The classical approaches to modeling and simulation in solid mechanics rely heavily on constitutive models. These provide constitutive equations, which complement the balance equations of field, or boundary- value, problems. Extensive ongoing research effortsare devoted in the scientific community to tune and ever improve constitutive models and equations for various classes of materials and various regimes of solicitation (loading amplitudes and rates, temperature, chemistry, ...), as well as to identify associated parameters. Constitutive models thus present a very large diversity encompassing a wide range of applications, yet this variety also hints at the inherent epistemic uncertainty carried by these models. If the uncertainty associated to constitutive parameters can be quantified, the uncertainty associated to the models themselves is much more difficult to measure. From a more historical point of view, constitutive models were initially conceived to generalize experimental observations made on specific (typically homogeneous) loading regimes to more general loadings.
With the recent progress in imaging techniques, experimental observations are nowadays much richer in information and existing constitutive models are sometimes incompatible with this abundance of data. Data-driven approaches have recently been developed to better exploit the large volumes of modern experimental measures, while attempting to avoid the bias induced by constitutive models.
The present course will focus on a global data-driven approach, completely avoiding the use of models (statistical models or constitutive models), which could thus be labelled as model-free.
The proposed course will constitute a consistent and comprehensive introduction to the model-free data-driven paradigm for computational solid mechanics. After a general introduction to the data-driven paradigm, and how it fundamentally differs from the classical paradigm, the course will take students all the way from acquiring rich mechanical data sets, notably from imaging, to data-driven numerical simulation in nonlinear mechanics of structures. On the way, important aspects such as mathematical foundations of data-driven and machine learning methods, and the necessity and ways to account for the stochastic and imperfect nature of real-life data will be covered. Abundant data are also generated in multi-scale approaches, and the course will discuss how the data-driven paradigm may be relevant in that context as well. Finally, the current challenges in dealing with non- linearities and history-dependent behaviors will be discussed.
The course will also include a series of practical hands-on sessions, where the students will experiment with the data-driven approach, starting from a series of images from which to extract data, process it to construct a material database, and use this database in a data-driven simulation. It will also be shown how to couple data- driven algorithms with standard finite element software such as Abaqus.
Kirchdoerfer T, Ortiz M (2016) Data- driven computational mechanics. Computer Methods in Applied Mechanics and Engineering 304:81-101, doi:10.1016/j. cma.2016.02.001.t
Kirchdoerfer T, Ortiz M (2017) Data-driven computing with noisy material data sets. Computer Methods in Applied Mechanics and Engineering 326:622-641, doi:10.1016/j.cma.2017.07.039.
Ayensa-Jiménez J, Doweidar MH, Sanz-Herrera JA, Doblaré M (2018) A new reliability-based data-driven approach for noisy experimental data with physical constraints. Computer Methods in Applied Mechanics and Engineering 328:752-774, doi:10.1016/j. cma.2017.08.027.
Conti S, Müller S, Ortiz M (2018) Data-driven problems in elasticity. Archives in Rational Mechanics and Analysis 229:79-123, doi:10.1007/ s00205-017-1214-0.
Eggersmann R, Stainier L, Ortiz M, Reese S (2021) Efficient data structures for model-free data- driven computational mechanics. Computer Methods in Applied Mechanics and Engineering 382:113855, doi:10.1016/j. cma.2021.113855.
Ayensa-Jiménez J, Doweidar MH, Sanz-Herrera JA, Doblaré M (2019) An unsupervised data completion method for physically-based data-driven models. Computer Methods in Applied Mechanics and Engineering 344:120-143, doi:10.1016/j.cma.2018.09.035.
Arridge S, Maass P, Öktem, O, Schönlieb CB (2019) Solving inverse problems using data-driven models. Acta Numerica 28:1-174, doi:10.1017/S0962492919000059.
Eggersmann R, Kirchdoerfer T, Reese S, Stainier L, Ortiz M (2019) Model-free data-driven inelasticity. Computer Methods in Applied Mechanics and Engineering 350:81- 99, doi:10.1016/j.cma.2019.02.016.
Leygue A, Seghir R, Réthoré J, Coret M, Verron E, Stainier L (2019) Non-parametric material state field extraction from full field measurements. Computational Mechanics, doi:10.1007/s00466- 019-01725-z.
Stainier L, Leygue A, Ortiz M (2019) Model-free data-driven methods in mechanics: material data identification and solvers. Computational Mechanics, doi:10.1007/s00466-019-01731-1.
5 lectures on: handling data with random properties in data-driven applications; using physical knowledge to improve data handling with example to data filling in missing data problems; introducing the concept of physically-informed machine learning with examples in the prediction of input-output relations in problems defined by PDE systems and in extracting internal state knowledge.
6 lectures on: data-driven computational mechanics (DDCM) in linear and non-linear elasticity, general principle, distance minimization algorithms, extension to dynamics, noisy data and maximum-entropy data-driven solver; results from mathematical analysis of DDCM, convergence properties; extension to history-dependent behaviors, representational paradigms for material history, examples.
5 lectures on: algorithmic strategies and data representation for history-dependent data-driven simulations; examples and illustration of the methodology for viscoelasticity and elasto-plasticity; new methods for distance-minimizing data-driven schemes; new efficient data structures and search algorithms; coupling with standard finite element software; comparison with classical solvers for inelastic problems.
6 lectures on: basics of digital image correlation (DIC) and its resolution; numerical algorithms for the optical flow problem; the uncertainty / resolution compromise and regularization techniques; Finite Element Model Updating (FEMU) methods; data-driven identification (DDI) approach, formulation and implementation; hands-on session in DIC and DDI with applications to linear and non-linear elasticity.
6 lectures on: inverse imaging problems; variational regularisation models and partial differential equations; sparsity-promoting regularisation and total variation; applications to tomography, image segmentation, motion estimation and image fusion; mathematical analysis and numerical algorithms; machine learning approaches such as learned iterative reconstruction and learned regularisers with deep neural networks.
6 lectures on: distance minimizing data-driven algorithms; integrated mechanical design approach combining DDI+DDCM; importance sampling issues; hands-on session (DDI based DDCM for nonlinear elasticity); data-driven solvers for finite elasticity; data-driven paradigm for multi-scale computations.
The course is offered in a hybrid format giving the possibility to attend the course also by remote (on Microsoft Teams platform). On-site places are limited and assigned on first come first served basis.
The registration fees are:
- On-site participation, 600.00 Euro + VAT* * where applicable (bank charges are not included) Italian VAT is 22%.
This fee includes a complimentary bag, five fixed menu buffet lunches, hot beverages, downloadable lecture notes.
Deadline for on-site application is September 10, 2022.
- Online participation, 250.00 Euro + VAT* * where applicable (bank charges are not included) Italian VAT is 22%.
This fee includes downloadable lecture notes.
Deadline for online application is September 28, 2022.
Application forms should be sent on-line through CISM website
A message of confirmation will be sent to accepted participants.
Upon request a limited number of on-site participants can be accommodated at CISM Guest House at the price of 30 Euro per person/night (mail to: foresteria@cism.it).
CANCELLATION POLICY
Applicants may cancel their registration and receive a full refund by notifying CISM Secretariat in writing (by email) no later than:
- September 10, 2022 for on-site participants (no refund after the deadline);
- September 28, 2022 for online participants (no refund after the deadline).
Cancellation requests received after these deadlines will be charged a 50.00 Euro handling fee. Incorrect payments are subject to Euro 50,00 handling fee.
GRANTS
A limited number of participants from universities and research centres who are not supported by their own institutions can request the waive of the registration fee and/or free lodging.
Requests should be sent to CISM Secretariat by August 15, 2022 along with the applicant's curriculum and a letter of recommendation by the head of the department or a supervisor confirming that the institute cannot provide funding. Preference will be given to applicants from countries that sponsor CISM.
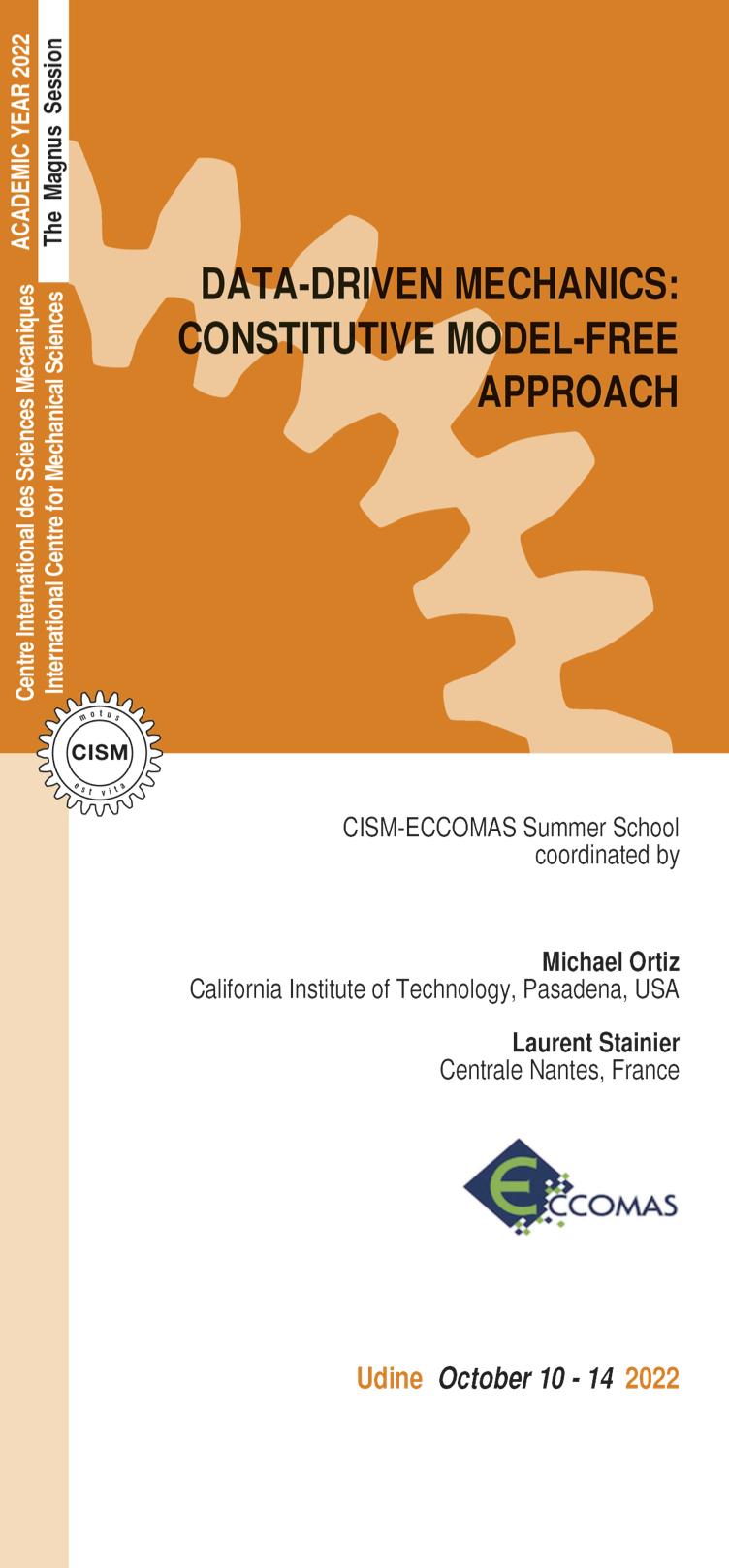